Examples of Hypergraph
We briefly introduce the fundamental properties, basic operations, and node classification task on hypergraph with EasyGraph.
Basic Properties and Operation of Hypergraph
Important
Each hyperedge in the hypergraph is an unordered set of vertices, which means that (0, 1, 2)
, (0, 2, 1)
, and (2, 1, 0)
are all the same hyperedge.
>>> import torch
>>> import easygraph as eg
>>> # Create a hypergraph with five nodes and three hyperedges.
>>> hg = eg.Hypergraph(5, [(0, 1, 2), (2, 3), (0, 4)])
>>> # print hyperedges with weights of hypergraph.
>>> hg.e
([(0, 1, 2), (2, 3), (0, 4)], [1.0, 1.0, 1.0])
>>> # print the incidence matrix of the hypergraph
>>> hg.H.to_dense()
tensor([[1., 0., 1.],
[1., 0., 0.],
[1., 1., 0.],
[0., 1., 0.],
[0., 0., 1.]])
>>> # Draw hypergraph with label of each vertex, color of vertexs and edges are set to blue and yellow respectively, line width of each hyperedges depends on its weight.
>>> hg.draw(v_label = [0,1,2,3,4], v_color = 'b', e_color = 'y', e_line_width = hg.e[1])
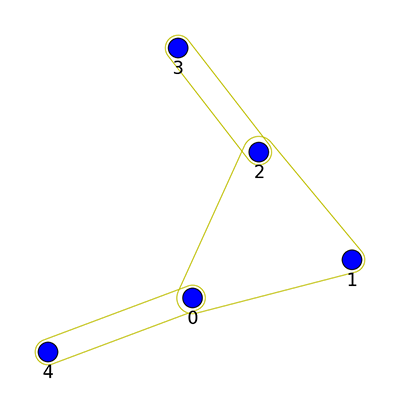
Add hyperedges and you can find the weight of the last hyperedge is 1.0 and 2.0, if you set the merge_op to mean and sum, respectively.
>>> hg = eg.Hypergraph(5, [(0, 1, 2), (2, 3), (2, 3), (0, 4)], merge_op="mean")
>>> hg.e
([(0, 1, 2), (2, 3), (0, 4)], [1.0, 1.0, 1.0])
>>> hg.add_hyperedges([(0, 2, 1), (0, 4)], merge_op="mean")
>>> hg.e
([(0, 1, 2), (2, 3), (0, 4), (2, 4)], [1.0, 2.0, 1.0, 1.0])
>>> hg.add_hyperedges([(2, 4)], merge_op="sum")
>>> hg.e
([(0, 1, 2), (2, 3), (0, 4)], [2.0, 2.0, 2.0])
>>> hg.remove_hyperedges([(2, 3)])
>>> hg.e
([(0, 1, 2), (0, 4), (2, 4)], [1.0, 1.0, 1.0])
Note
If the added hyperedges have duplicate hyperedges, those duplicate hyperedges will be automatically merged with specified merge_op. If merge_op = ‘sum’, the weight is the sum of duplicate hyperedges weights. If merge_op = ‘mean’, the weight is the average of sum of duplicate hyperedges weights.
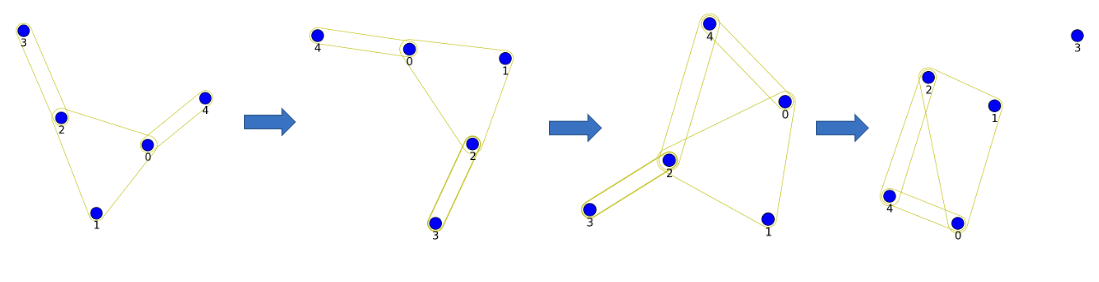
Create a hypergraph based on the k-nearest neighbors of the features.
>>> X = torch.tensor([[0.0658, 0.3191, 0.0204, 0.6955],
[0.1144, 0.7131, 0.3643, 0.4707],
[0.2250, 0.0620, 0.0379, 0.2848],
[0.0619, 0.4898, 0.9368, 0.7433],
[0.5380, 0.3119, 0.6462, 0.4311]])
>>> hg = eg.Hypergraph.from_feature_kNN(X, k=3)
>>> hg
Hypergraph(num_v=5, num_e=4)
>>> hg.e
([(0, 1, 2), (0, 1, 4), (0, 2, 4), (1, 3, 4)], [1.0, 1.0, 1.0, 1.0])
>>> hg.H.to_dense()
tensor([[1., 1., 1., 0.],
[1., 1., 0., 1.],
[1., 0., 1., 0.],
[0., 0., 0., 1.],
[0., 1., 1., 1.]])
>>> hg.draw(v_label=list(range(0,10)))
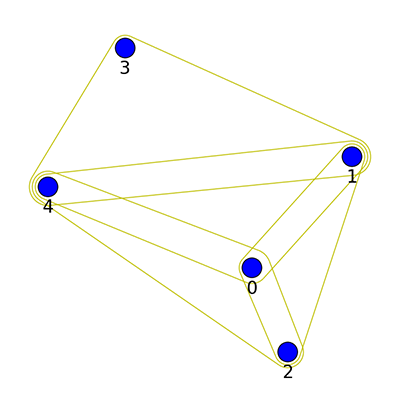
Construct a hypergraph from a graph.
>>> g = eg.Graph()
>>> g.add_edges([(0, 1), (1, 2), (2, 3), (1, 4)])
>>> hg = eg.Hypergraph.from_graph(g)
>>> hg.e
([(0, 1), (1, 2), (1, 4), (2, 3)], [1.0, 1.0, 1.0, 1.0])
>>> hg.H.to_dense()
tensor([[1., 0., 0., 0.],
[1., 1., 1., 0.],
[0., 1., 0., 1.],
[0., 0., 0., 1.],
[0., 0., 1., 0.]])
Train a Hypergraph model, HGNN+ on Cooking200
We present a specifical node classification task on Cora with a hypergraph neural network HGNN++
Model:
HGNN+ (eg.models.HGNNP): HGNN+: General Hypergraph Neural Networks paper (IEEE T-PAMI 2022).
Dataset:
Cooking 200 (eg.data.Cooking200): A cooking recipe hypergraph dataset collected from Yummly.com for vertex classification task
Import Libraries
>>> import torch
>>> import torch.nn as nn
>>> import torch.optim as optim
>>> from easygraph import Hypergraph
>>> from easygraph.datasets import Cooking200
>>> from easygraph import HGNNP
>>> from easygraph import set_seed
>>> from easygraph.experiments import HypergraphVertexClassificationTask as Task
>>> from easygraph.ml_metrics import HypergraphVertexClassificationEvaluator as Evaluator
Define Functions
>>> def structure_builder(trial):
>>> global hg_base, g
>>> cur_hg: Hypergraph = hg_base.clone()
>>> return cur_hg
>>>
>>> def model_builder(trial):
>>> return HGNNP(dim_features, trial.suggest_int("hidden_dim", 10, 20), num_classes, use_bn=True
Main
>>> if __name__ == "__main__":
>>> work_root = "/Users/yizhihenpidehou/Desktop/tmp"
>>> set_seed(2022)
>>> device = torch.device("cuda") if torch.cuda.is_available() else torch.device("cpu")
>>> data = Cooking200()
>>> data
This is cooking_200 dataset:
-> num_classes
-> num_vertices
-> num_edges
-> edge_list
-> labels
-> train_mask
-> val_mask
-> test_mask
Please try `data['name']` to get the specified data.
>>> dim_features = data["num_vertices"]
>>> num_classes = data["num_classes"]
>>> hg_base = Hypergraph(data["num_vertices"], data["edge_list"])
>>> input_data = {
"features": torch.eye(data["num_vertices"]),
"labels": data["labels"],
"train_mask": data["train_mask"],
"val_mask": data["val_mask"],
"test_mask": data["test_mask"],
}
>>> evaluator = Evaluator(["accuracy", "f1_score", {"f1_score": {"average": "micro"}}])
>>> task = Task(
work_root, input_data, model_builder, train_builder, evaluator, device, structure_builder=structure_builder,
)
>>> task.run(200, 50, "maximize")
Outputs
[I 2023-01-30 17:17:02,268] Logs will be saved to /Users/yizhihenpidehou/Desktop/tmp/2023-01-30--17-17-02/log.txt
[I 2023-01-30 17:17:02,269] Files in training will be saved in /Users/yizhihenpidehou/Desktop/tmp/2023-01-30--17-17-02
[I 2023-01-30 17:17:02,269] Random seed is 1675070222
[I 2023-01-30 17:17:02,270] A new study created in memory with name: no-name-ec259b1d-f2c9-438b-9fc8-7efab4ee2b1b
/Users/yizhihenpidehou/Desktop/fdu/eg/Easy-Graph/train_gnn.py:27: FutureWarning: suggest_loguniform has been deprecated in v3.0.0. This feature will be removed in v6.0.0. See https://github.com/optuna/optuna/releases/tag/v3.0.0. Use :func:`~optuna.trial.Trial.suggest_float` instead.
lr=trial.suggest_loguniform("lr", 1e-4, 1e-2),
/Users/yizhihenpidehou/Desktop/fdu/eg/Easy-Graph/train_gnn.py:28: FutureWarning: suggest_loguniform has been deprecated in v3.0.0. This feature will be removed in v6.0.0. See https://github.com/optuna/optuna/releases/tag/v3.0.0. Use :func:`~optuna.trial.Trial.suggest_float` instead.
weight_decay=trial.suggest_loguniform("weight_decay", 1e-4, 1e-2),
[I 2023-01-30 17:17:20,571] Trial 0 finished with value: 0.4699999988079071 and parameters: {'hidden_dim': 19, 'lr': 0.00011393568005854129, 'weight_decay': 0.000260717506361872}. Best is trial 0 with value: 0.4699999988079071.
[I 2023-01-30 17:17:36,173] Trial 1 finished with value: 0.48500001430511475 and parameters: {'hidden_dim': 20, 'lr': 0.0004400638127040677, 'weight_decay': 0.0024733110034356118}. Best is trial 1 with value: 0.48500001430511475.
[I 2023-01-30 17:17:51,809] Trial 2 finished with value: 0.5049999952316284 and parameters: {'hidden_dim': 20, 'lr': 0.002990964903897353, 'weight_decay': 0.003064446178951424}. Best is trial 2 with value: 0.5049999952316284.
[I 2023-01-30 17:18:07,705] Trial 3 finished with value: 0.4749999940395355 and parameters: {'hidden_dim': 14, 'lr': 0.005141482540718165, 'weight_decay': 0.006598448985658328}. Best is trial 2 with value: 0.5049999952316284.
[I 2023-01-30 17:18:23,885] Trial 4 finished with value: 0.4300000071525574 and parameters: {'hidden_dim': 15, 'lr': 0.0002248879282427639, 'weight_decay': 0.0044752065322836545}. Best is trial 2 with value: 0.5049999952316284.
[I 2023-01-30 17:18:31,593] Trial 5 pruned.
[I 2023-01-30 17:18:36,038] Trial 6 pruned.
[I 2023-01-30 17:18:39,870] Trial 7 pruned.
[I 2023-01-30 17:18:48,675] Trial 8 pruned.
[I 2023-01-30 17:18:53,612] Trial 9 pruned.
[I 2023-01-30 17:18:57,210] Trial 10 pruned.
[I 2023-01-30 17:19:05,217] Trial 11 pruned.
[I 2023-01-30 17:19:13,432] Trial 12 pruned.
[I 2023-01-30 17:19:18,591] Trial 13 pruned.
[I 2023-01-30 17:19:34,381] Trial 14 finished with value: 0.47999998927116394 and parameters: {'hidden_dim': 20, 'lr': 0.005628442102091454, 'weight_decay': 0.0027947441404729202}. Best is trial 2 with value: 0.5049999952316284.
[I 2023-01-30 17:19:42,771] Trial 15 pruned.
[I 2023-01-30 17:19:47,172] Trial 16 pruned.
[I 2023-01-30 17:19:50,671] Trial 17 pruned.
[I 2023-01-30 17:19:54,735] Trial 18 pruned.
[I 2023-01-30 17:19:59,581] Trial 19 pruned.
[I 2023-01-30 17:20:17,514] Trial 20 finished with value: 0.4950000047683716 and parameters: {'hidden_dim': 18, 'lr': 0.001047739904975789, 'weight_decay': 0.00456408904231232}. Best is trial 2 with value: 0.5049999952316284.
[I 2023-01-30 17:20:22,516] Trial 21 pruned.
[I 2023-01-30 17:20:28,277] Trial 22 pruned.
[I 2023-01-30 17:20:32,122] Trial 23 pruned.
[I 2023-01-30 17:20:36,993] Trial 24 pruned.
[I 2023-01-30 17:20:42,132] Trial 25 pruned.
[I 2023-01-30 17:20:56,416] Trial 26 finished with value: 0.4749999940395355 and parameters: {'hidden_dim': 16, 'lr': 0.005446543934019758, 'weight_decay': 0.0009659159646018803}. Best is trial 2 with value: 0.5049999952316284.
[I 2023-01-30 17:21:01,049] Trial 27 pruned.
[I 2023-01-30 17:21:05,123] Trial 28 pruned.
[I 2023-01-30 17:21:08,891] Trial 29 pruned.
[I 2023-01-30 17:21:23,526] Trial 30 finished with value: 0.5 and parameters: {'hidden_dim': 16, 'lr': 0.003970445142112136, 'weight_decay': 0.0010214619854412256}. Best is trial 2 with value: 0.5049999952316284.
[I 2023-01-30 17:21:28,012] Trial 31 pruned.
[I 2023-01-30 17:21:45,132] Trial 32 finished with value: 0.4950000047683716 and parameters: {'hidden_dim': 17, 'lr': 0.009314593992038237, 'weight_decay': 0.00046271963422662684}. Best is trial 2 with value: 0.5049999952316284.
[I 2023-01-30 17:22:02,096] Trial 33 finished with value: 0.48500001430511475 and parameters: {'hidden_dim': 17, 'lr': 0.009337061774729634, 'weight_decay': 0.0004076408890486919}. Best is trial 2 with value: 0.5049999952316284.
[I 2023-01-30 17:22:18,922] Trial 34 finished with value: 0.5149999856948853 and parameters: {'hidden_dim': 15, 'lr': 0.007286750524788286, 'weight_decay': 0.0002458354082341516}. Best is trial 34 with value: 0.5149999856948853.
[I 2023-01-30 17:22:26,266] Trial 35 pruned.
[I 2023-01-30 17:22:31,649] Trial 36 pruned.
[I 2023-01-30 17:22:45,710] Trial 37 finished with value: 0.4950000047683716 and parameters: {'hidden_dim': 16, 'lr': 0.0043213824761157175, 'weight_decay': 0.0002711486260724712}. Best is trial 34 with value: 0.5149999856948853.
[I 2023-01-30 17:22:49,650] Trial 38 pruned.
[I 2023-01-30 17:23:06,202] Trial 39 finished with value: 0.47999998927116394 and parameters: {'hidden_dim': 15, 'lr': 0.007639715234791997, 'weight_decay': 0.0005264771015557918}. Best is trial 34 with value: 0.5149999856948853.
[I 2023-01-30 17:23:10,989] Trial 40 pruned.
[I 2023-01-30 17:23:15,114] Trial 41 pruned.
[I 2023-01-30 17:23:22,072] Trial 42 pruned.
[I 2023-01-30 17:23:39,965] Trial 43 finished with value: 0.4950000047683716 and parameters: {'hidden_dim': 17, 'lr': 0.00932715468988115, 'weight_decay': 0.0004701066750730449}. Best is trial 34 with value: 0.5149999856948853.
[I 2023-01-30 17:23:56,860] Trial 44 finished with value: 0.5149999856948853 and parameters: {'hidden_dim': 16, 'lr': 0.004861316886763922, 'weight_decay': 0.00017801679034748196}. Best is trial 34 with value: 0.5149999856948853.
[I 2023-01-30 17:24:02,154] Trial 45 pruned.
[I 2023-01-30 17:24:06,111] Trial 46 pruned.
[I 2023-01-30 17:24:09,757] Trial 47 pruned.
[I 2023-01-30 17:24:26,617] Trial 48 finished with value: 0.47999998927116394 and parameters: {'hidden_dim': 15, 'lr': 0.00996806266543509, 'weight_decay': 0.0005849276525444326}. Best is trial 34 with value: 0.5149999856948853.
[I 2023-01-30 17:24:32,282] Trial 49 pruned.
[I 2023-01-30 17:24:32,360] Best trial:
[I 2023-01-30 17:24:32,361] Value: 0.515
[I 2023-01-30 17:24:32,361] Params:
[I 2023-01-30 17:24:32,361] hidden_dim |-> 15
[I 2023-01-30 17:24:32,361] lr |-> 0.007286750524788286
[I 2023-01-30 17:24:32,361] weight_decay |-> 0.0002458354082341516
[I 2023-01-30 17:24:32,412] Final test results:
[I 2023-01-30 17:24:32,412] accuracy |-> 0.531
[I 2023-01-30 17:24:32,412] f1_score |-> 0.395
[I 2023-01-30 17:24:32,412] f1_score -> average@micro |-> 0.531